Spatial distribution maps Spatial distribution maps of trends were created in SURFER using kriging methods to illustrate the study variables. Exponential variograms were used to generate maps. Municipalities were assessed as single points, rather than areas of land. So to identify spatial trends, we focused on groups of municipalities, such as departments. Post maps of significant Moran’s I values were overlaid on the maps.
RESULTS
GWR All independent variables produced significantly correlated models with both unCKD and ndESRD hospital admission rates separately (p < 0.001, Table 1), except for unCKD admission and percent area of sorghum cultivation. Mean maximum ambient temperature was used to represent temperature variation in multivariate regression models because it correlated more strongly with unCKD and ndESRD hospital admission rates than did mean average ambient temperature
Table 1: unCKD hospital admissions ptp and environmental parameters (n = 259 municipalities); and ndESRD and environmental parameters (n = 242 municipalities), multiple stepwise geographically-weighted regression analysis
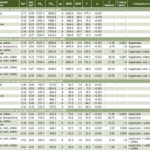
Adj. Rp2 : adjusted Rp2 (if no change or lower, adding variable does not improve model)
MSD: mean square of the deviation
MSR: mean square of the regression
ndESRD: nondiabetic end-stage renal disease
np: number of environmental parameters in model
ptp: per ten thousand population
Rp2: coefficient of multiple determination, higher number means better model
SSD: sum of squares of the residual
SSR: sum of squares of the regression
unCKD: unspecified chronic kidney disease
Percent area of sugarcane cultivation produced the greatest bivariate regressions (Rp2 = 0.77 and 0.48 for unCKD and ndESRD, respectively). Therefore, these models were used as restricted bases in the F tests to verify significance of adding other parameters. In the unCKD model set, the most significantly predictive regression model included percent area of sugarcane, cotton and corn cultivation (Rp2 = 0.80, Table 1). Thus, 80% of the variation in unCKD hospital admission distribution is reflected by proportional variation of these three crops. Multivariate models for ndESRD admission rates had much lower coefficients of determination, with the most significant model producing Rp2 = 0.52 for percent area of sugarcane, corn, cotton and coffee cultivation (Table 1). Percent areas of bean and sorghum cultivation did not significantly improve fit for either model, according to relatively high F test p values. For unCKD and ndESRD, adding the average maximum temperature as a regressor variable did not significantly improve the model.
Distribution Maps and Moran’s I The areas of highest unCKD admission rates were located in the southwestern municipalities of La Paz Department (up to 77.4 ptp), southern San Salvador Department (up to 14.4 ptp), and southeastern La Libertad Department (up to 11.4 ptp) (Figure 1a). Dots in the Figures represent clusters determined with the local Moran’s I. Regions with greatest percent areas of sugarcane cultivation were in northern San Salvador (up to 49.3%), southwestern and central La Paz (up to 38.1%), northeastern San Vicente (37.4%), and central Sonsonate (37.1%) (Figure 1b). Percent area of cotton cultivation was greatest in west-central La Paz (up to 1.96%), central San Miguel (up to 1.7%) and southern Usulután (up to 1.65%) (Figure 1c). Highest temperatures were in the southeastern municipalities of San Miguel (up to 35.3 ºC) and La Union (up to 35.2 ºC) (Figure 1d). The map for ndESRD admissions is similar to that for unCKD hospital admissions and is available online in Supporting Information, as well as maps of percent area corn, coffee, bean and sorghum cultivation; and mean average ambient temperature (Figures S1–6, available online at www.medicc.org/mediccreview/VanDervort_Fig)
Figure 1: Clusteringa of unCKD by municipalityb, sugarcane and cotton cultivationc, and temperatured
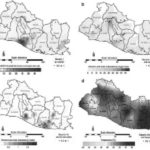
a univariate local Moran’s I values for positive unCKD clustering; only clusters with Moran’s I ≥0.3
and p < 0.05 are plotted
b municipal rates/10,000 population 2006–2010
c % of area cultivating each crop in 2007–2008
d mean maximum ambient temperature 1970–2000
(figures in color available at www.medicc.org/mediccreview/VanDervort_Fig)
Areas with largest percent area of sugarcane and cotton cultivation were similar to areas with highest unCKD admission rates. However, there was an area of relatively high unCKD hospital admission rates in southeastern El Salvador that was not reflected in percent area of sugarcane cultivation. This area was in the region of highest ambient temperatures (33–36 ºC), corresponding to La Unión and San Miguel states (Figure 1d).
Global univariate Moran’s I was 0.20 for unCKD and 0.33 for ndESRD, which indicates some degree of clustering. Global bivariate Moran’s I for unCKD association with percent area of sugarcane, cotton and corn cultivation; and mean maximum temperature were 0.11, 0.22, 0.11, and 0.12, respectively. For ndESRD, Moran’s I was 0.12, 0.21, 0.09, and 0.24, respectively for the same variables. Figures 1a–d also show post maps of local univariate and bivariate Moran’s I values of 0.3–1.0 (p ≤0.05). Moran’s I values in Figure 1a identify clustering of unCKD admissions; those in Figures 1b–d show positive clustering for unCKD hospital admission rates with percent area of sugarcane and cotton cultivation, and mean maximum ambient temperature, respectively. Focusing on Moran’s I values with the greatest positive response for clustering (I = 0.3–1.0), the clusters of unCKD hospital admission rates were located near the regions of highest values of the unCKD hospital admission rate variable. Moran’s I values of greatest positive response for clustering were near areas of largest percent area of sugarcane and cotton cultivation. The clustering pattern was different, however, in Figure 1d, where the greatest positive response for clustering values did not consistently plot near the highest temperatures; there were some positive clusters in regions of lower temperatures. Clusters of ndESRD were also observed (Figure S1 in Supporting Information), with some occurring in areas of relatively low CKD incidence in western El Salvador. Note that some clusters are a little offset with respect to the maximum in the graph. From the definition of Moran’s I, the index depends not only on the value at the point but also on the values of neighboring points. For bivariate local Moran’s I, the value depends on two variables (e.g., unCKD and percent area of sugarcane) which can also produce some offset.
DISCUSSION
Bivariate GWR found the principal parameter predicting municipal unCKD hospital admission rates was percent area of sugarcane cultivation, followed by mean maximum ambient temperature, percent cotton cultivation and percent corn cultivation. This suggests that proximity to agriculture (especially sugarcane) along with high ambient temperatures may influence the rate of unCKD in El Salvador.
Stepwise GWR showed that unCKD hospital admission rates are best predicted by percent area of sugarcane, cotton and corn cultivation; percent area of sorghum, beans, or coffee, and mean maximum ambient temperature did not improve the model. These differences could be explained by the distributions of the specific crops cultivated. Coffee is usually cultivated in high regions of El Salvador’s volcanic chain. Beans and sorghum are cultivated usually in small plots of land, while sugarcane and cotton are cultivated on large tracts. Corn is the most commonly cultivated crop in El Salvador. The amount and variety of agrochemicals applied to specific crops could also explain the GWR results, if more harmful chemicals or greater amounts of them are applied to sugarcane, cotton and corn fields. This needs to be assessed and quantified. Many pesticides with paraquat, glyphosate, and 2,4-D as active ingredients have been restricted in some developed countries,[43] but are still in use in El Salvador and other countries, without adequate understanding of exposure consequences. Assuming that agrochemicals are applied to agricultural fields in El Salvador (a plausible assumption, given the amount of pesticides imported), the associated toxins could be compromising kidney health, especially if there is substantial physical contact.
In 2011, Bajo Lempa farmers with CKDu were interviewed about their agrochemical use.[44] Results showed potential CKDu causes in this population were unsafe and prolonged use of agrochemicals and underuse of personal protective equipment. Accumulation of persistent contaminants in soil, water, or atmosphere may also increase threat to health. Occupational exposure may explain the sex difference in CKDu prevalence in Bajo Lempa in 2009 (25.7% in men and 11.8% in women).[18] However, without knowing the specific toxin and exposure route, it is difficult to characterize the male:female imbalance.
In another study in El Salvador, a high prevalence of CKD was found in men living near the hotter coast (18%) than in the cooler highlands (1%).[15] Statistical analysis did not reveal significant differences between sugarcane work and other occupations, which is not consistent with our results. However, in that study the districts located at higher altitudes did not have a high density of sugarcane cultivation, and thus may not have had the same degree of exposure to agricultural toxins.
Cadmium is one of the most nephrotoxic heavy metals. It can produce tubular damage,[45,46] through oxidative stress from unbalanced production of reactive oxygen species, possibly due to cadmium-induced abnormalities in cell mitochondria.[47] In Sri Lanka, CKD of unknown etiology is thought to be caused by cadmium ingestion which is accelerated by in situ fluoride in drinking water.[48] The cadmium source was triple superphosphate fertilizer with 23.5–71.7 mg Cd/kg, which accumulated in river sediment.[48,49] Cadmium concentrations in soil increase with fertilizer application rate and the metal can accumulate in crops grown in soils in which the fertilizer is applied.[50]
In another Sri Lankan study, a relationship was suggested between epidemic kidney disease and consumption of aqueous fluoride and arsenic in hard, alkaline water.[51] In that research, 68% of CKDu patients and only 28% of controls had urine arsenic levels higher than 21 µg/g creatinine (the putative threshold for early changes in the kidney). In addition, 48% of CKDu patients versus only 17.4% of controls were above the threshold level for chronic arsenic toxicity. Other nephrotoxins, such as NSAIDs[7] and consumption of unregulated alcohol[22] have also been proposed as causal factors for CKDu.
The addition of mean maximum ambient temperature did not improve the regression, suggesting that it is not a main factor. This is also confirmed in the spatial distribution maps, showing that areas with higher ndCKD hospital admission rates (Figure 1a) do not correspond with areas of higher mean maximum ambient temperature (Figure 1d). Laborers in Central America have been working in high ambient temperature conditions for hundreds of years. Ambient temperatures on the Pacific coast of western Costa Rica, Nicaragua and southern Honduras are as high or higher than maximum temperatures in El Salvador,[52] but CKDu was not observed in previous generations. High temperatures may be an indirect or potentiating factor, as people need to consume larger quantities of water in hotter climates to account for loss of fluids from perspiration. If available drinking water contains nephrotoxins (i.e., naturally occurring heavy metals or other nephrotoxic compounds), then larger doses may be consumed in hotter regions, influencing kidney health.[4] If they do not consume enough water to compensate for volume depletion, then the concentration of any nephrotoxic compounds would increase in their bodies.
The unCKD and ndESRD data we used were only from public hospitals and clinics. In El Salvador, the majority of the population (~80%) depends on the public health system for medical attention, because of high poverty levels.[53] Only one fifth of the population has access to social security hospitals (affiliated to the national system of health and pension). However, as unCKD is associated with low socioeconomic status,[6] public hospitals may account for an even greater share of unCKD hospital admissions.
A limitation of the study is that data originally tagged “unidentified CKD” might have included some cases with diabetes identified later, which could generate some bias toward the null. This was not true for ndESRD data. The study was also limited by using environmental variables that may be indirectly related to unCKD in El Salvador, and nephrotoxin exposure route is not addressed. This preliminary assessment of the relationships between the disease and broad variables was meant to help determine the most efficient investigative plan. CKDu is a problem seen not only in Central America, but in other countries around the world. It is imperative to continue researching CKDu causes by analyzing water, soil, and produce from sugarcane, cotton, and corn fields, as well as human blood samples to detect toxins. It is also important to analyze the various fertilizers and pesticides sold in El Salvador and other regions with high CKDu occurrence, to determine the composition of heavy metals or other impurities. The route of exposure will then need to be identified to prevent further kidney damage. Meanwhile, our research suggests that regulatory agencies address control of import, sale and application of pesticides and fertilizers, to protect the environment and population.
CONCLUSIONS
High temperatures do not appear to strongly influence occurrence of unCKDu proxies. CKDu in El Salvador may arise from proximity to agriculture to which agrochemicals are applied, especially in sugarcane cultivation. The findings of this preliminary ecological study suggest that more research is needed to assess and quantify presence of specific agrochemicals in high-CKDu areas.
ACKNOWLEDGMENTS
We are indebted to MINSAL, MARN and El Salvador’s Ministry of Agriculture and Livestock Farming for providing data. We thank Dr Paulo Ortiz for assistance with statistical analysis.