INTRODUCTION
Early assessment of risk of death or complications in patients undergoing major abdominal surgery (MAS) is imperative in clinical practice. Assessing prognosis enables better organization of available resources and is useful for establishing benchmarks for improving health care quality. The actual number of deaths should be close to the estimated number, or decrease as quality of care increases. Assessing prognosis is thus a useful resource for evaluation and auditing.[1] A large deviation from the predicted standard may indicate a failure in one or more steps in the health care process.
Assessing prognosis supports treatment strategies for patients who have required MAS, helping define the best options for decreasing risk of complications and death. This is especially true for patients with intra-abdominal infectious complications (IIC) requiring reoperation.[2,3] Early detection of complications notably influences improvement in outcomes.[1,4–7] However, in these patients, prognosis tends to be difficult to assess,[4] which carries the risk of a late decision to reoperate.[2,8] Most surgeons’ criteria are based on clinical practice, personal experience and diagnostic tests, but this approach to decision-making is skills-based and therefore its reliability varies among professionals. It has been argued that routine clinical and paraclinical parameters have limited value for early IIC diagnosis.[6]
Axial tomography is the gold standard among diagnostic imaging methods for IIC detection, but the technology may not always be available, and it requires hemodynamic stability.[3]
Abdominal ultrasound also has limitations in the presence of abdominal distension, intense pain, or intestinal gas.[3] More recently, biomarkers such as procalcitonin, C-reactive protein and some cytokines (such as interleukin 6 and tumor necrosis factor) have been reported useful in IIC diagnosis,[5,6,9] but data on their diagnostic or prognostic value have been inconsistent.[5,6] Furthermore, systematic measurement of biomarkers could increase cost of care,[6] an additional obstacle to their use in ICUs with limited budgets and resources.
Scoring systems are instruments validated by clinical research to support medical decision-making. Their advantage lies in contributing objectivity to assessment of patient status, but they are not employed systematically in all services. IIC-risk scoring systems are mainly used for comparing research results and as audit tools.[1,7] While it is hoped that they will be able to guide clinical decisions in future, they are not currently considered reliable for gauging individual prognosis.[1,7]
Among scoring systems for peritonitis the Manheim peritonitis index and the Altona index are still in use as indicators of severity in cases of secondary bacterial peritonitis.[1,7] They enable appropriate classification of patients and comparison of those from different institutions.[10,11] More frequently used are nonspecific scoring systems for MAS, such as the Acute Physiology and Chronic Health Evaluation II (APACHE II), which is calculated within 24 hours of admission. It is widely used in intensive care and includes 12 physiological variables and laboratory tests not always available in all ICUs.[12] Additionally, its application is limited in assessing the effects of interventions on physiological variables in patients with peritonitis.[7]
According to Ramsay, an ideal scoring system should quantify severity of surgical patients’ risk of death or major complications, based on readily available objective data, and in a timely manner. It should be easy to use, clearly define the condition to be predicted, and be applicable for audit purposes.[13] Therefore, it would be useful to create prognostic scoring systems based on simple indicators routinely applicable in health services.
Several factors are involved in post-MAS clinical course whose relationship to mortality justifies their inclusion in scoring systems: age, malignancy (as a cause or comorbidity), acute impairment of vital physiological variables, failure to control focus of infection, and nutritional status,[7,14–22] Elevated intra-abdominal pressure (IAP) is an independent factor related to complications and death after MAS.[23–32] The fact that its measurement does not require major resources suggests it would be particularly useful in low-resource settings, but to date there have been no reports of its inclusion in predictive indices of mortality after MAS.
In short, there is no optimal scoring system for comprehensive assessment of individual patients after MAS. The objective of this study is to assess the utility of a model based on IAP for predicting death after MAS.
METHODS
Design and population A prospective observational cohort study was done from January 2008 through January 2010, with 389 patients in the period immediately following emergency or elective abdominal surgery, who had been admitted consecutively to the ICU of a university hospital connected to the General Calixto García Medical Faculty. Patients who remained in the ICU for ≥48 hours were included. Excluded patients comprised 21 who died in the first 48 hours following surgery, 7 patients in whom transvesical IAP could not be measured (among them, 5 cases of pregnancy), 33 patients reoperated for noninfectious causes (because of their low influence on mortality in the postoperative period following MAS) and 28 patients with scheduled reoperations (due to the extent of their peritonitis, prognosis was already known to be poor). The final sample included 300 patients, who were assigned by simple random sampling to test and validation groups.
Variables The dependent variable was patient condition (alive or deceased) on discharge. Independent variables, selected for their association with MAS complications and mortality widely reported in the literature,[10–19,22–32] were:
- APACHE II, calculated by weighing results of variables that identify vital physiological impairment, from both physical examination (rectal temperature, mean arterial pressure, heart rate, respiratory rate) and ancillary tests (alveolar-arterial oxygen difference as fraction of inspired oxygen, arterial pH, serum sodium, serum potassium, creatinine, hematocrit and white blood count), added to points assigned for age and chronic disease history;[12]
- age in completed years (included in APACHE II but with a low correlation, <0.6, and we were particularly interested in assessing its value as an independent factor);
- sex;
- presence of malignancy (reported or confirmed); and
- IAP (mmHg).
Data collection Pressure measurement (e.g., arterial blood pressure, central venous pressure) is an essential part of monitoring vital signs in ICU. It is a routine and simple practice in any ICU, commonly using a transducer with a graduated scale in centimeters of water, as for central venous pressure. To measure IAP in this study, instead of using a pressure transducer, a column of water with a centimeter scale was placed in the urine drainage system, using the technique described by Cheatham and Safcsak,[33] following recommendations by The Abdominal Compartment Society.[25] Two end-expiratory measurements were taken, six hours apart, within 24 hours of admission.
To control for measurement biases, ICU nurses were trained in the technique, which was duly standardized and always performed under specialist supervision. An intravesicular volume of 25 mL of saline solution was used in taking the measurement. Zero was placed at the axillary midline with the patient supine, using the superior iliac crest as a reference point.[25] Each IAP value was obtained via manometry (cmH2O) and recalculated as mmHg (1 cmH2O = 0.74 mmHg). The four values obtained for each patient were averaged and introduced into a database. Each patient was individually followed until hospital discharge (alive or deceased).
Data management and analysis For each quantitative variable (age, APACHE II and IAP) central tendency and dispersion measures were median and interquartile range, while frequencies and percentages were used for categorical variables (sex and malignancy). To verify between-groups similarity in categorical variables, the chi-square test with Yates correction for continuity was used, and the Fisher exact test when 25% or more of expected frequencies were <5. The Mann–Whitney U test was used to compare differences in continuous variable medians among groups under basal conditions, since they were not normally distributed. Parameters of the logistic regression model were estimated in the test group and predictive capacity of the models for new patients was assessed in the validation group.
Three logistic regression models were fitted for predicting probability of death:
- Model I (APACHE II+IAP): mortality by age, sex, malignancy, APACHE II and IAP.
- Model II (APACHE II): mortality by age, sex, malignancy and APACHE II.
- Model III (IAP): mortality by age, sex, malignancy and IAP.
To assess the magnitude of associations, odds ratios (OR) and their 95% confidence intervals (CI) were calculated. The receiver operating characteristic (ROC) curve was used to assess the capacity of each model to discriminate between living and deceased patients in each group, taking into account sensitivity and specificity values for each point. The discriminative power of the model was considered excellent if the area under the curve (AUC) was >0.80, very good if it was >0.75, and good if it was >0.70.[34] Calibration of each model was assessed using the Hosmer–Lemeshow goodness-of-fit test, according to which, p >0.05 indicates acceptable model calibration.[35] ROC AUCs were compared using the chi-square test of homogeneity.
SPSS 11.5 was used for data organization, validation and analysis.
Ethics The protocol was approved by the General Calixto García Medical Faculty Ethics Committee. After receiving information about the study, patients or next-of-kin (of unconscious patients) provided written informed consent before inclusion in the study. IAP measurement did not interfere with other diagnostic or therapeutic procedures, in accordance with the Helsinki Declaration.[36]
RESULTS
Table 1 displays the general characteristics of the study sample by vital status at discharge. There were significant differences between alive and deceased patients according to sex, age, reoperation, IAP and APACHE II score.
Influence of model variables on probability of dying is shown in Table 2. According to OR and p values, age was the variable with the most influence on probability of dying for the three models, APACHE II and IAP.
Table 3 displays validation of the three models, with practically identical results in the test and validation groups, with very good calibration (Hosmer–Lemeshow p >0.05) and discrimination (AUC close to unity) and no significant differences in discrimination among the three models. The similarity between the test and validation groups is evidence of external validity.
DISCUSSION
Three models were developed and validated to predict mortality in post–abdominal-surgery patients admitted to the ICU. The three models demonstrate similar capacity to discriminate between survivors and nonsurvivors. AUC values were so close that no one model could be deemed superior to the others.
From a clinical point of view, IAP’s contribution to the predictive value of the models containing it (IAP and APACHE II+IAP) is of great importance. IAP increases in physiopathological conditions and is 5–7 mmHg in critical patients.[25] A sustained increase in IAP during the first postoperative week leads to organ and system dysfunction, which is significantly associated with higher mortality.[17,37–39] Its effects on splanchnic circulation and on intra-abdominal organ function lead to progressive failure of renal, cardiorespiratory and hepatic function.[30,31,40,41] Intestinal mucosal damage due to mesenteric ischemia resulting from intra-abdominal hypertension leads to bacterial translocation and triggers multiple organ failure.[30,31,39–43] IAP magnitude reflects the cumulative effect of physiopathological impairments occurring after MAS.[23,24,37] For this reason, IAP adds specificity to the model and makes it recommendable for prediction of postoperative death in MAS patients, even though on its own, it is not comprehensive.
The IAP model was comparable in effectiveness to the one based on APACHE II, currently the most used scoring system for overall assessment of severity in critical patients.[16,18,37] As a predictive index, it enables assessment of extent of acute physiological impairment and weighs the influence of chronic diseases on patient status.[12] Its limitations are that it is not specific to surgical patients, that it only uses information from the first 24 hours after admission, and that it does not consider response to therapy.[12,44]
Table 1: Characteristics of surgical patients by discharge vital status
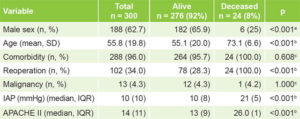
a two-tailed chi-square test with Yates correction b Mann–Whitney U test c Fisher exact test APACHE II: Acute Physiology and Chronic Health Evaluation II IAP: intra-abdominal pressure IQR: interquartile range
Table 2: Probability of dying by study variable
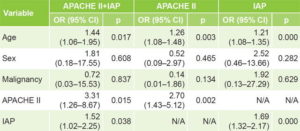
APACHE II: Acute Physiology and Chronic Health Evaluation II IAP: intra-abdominal pressure N/A: not applicable
Table 3: Model validation
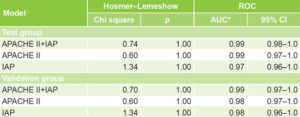
*chi square 2.80, df = 2, p = 0.094 APACHE II: Acute Physiology and Chronic Health Evaluation II AUC: area under the curve IAP: intra-abdominal pressure ROC: receiver operating characteristic
IAP is an independent risk factor for surgical patient mortality, as has been demonstrated in a variety of studies, and can be measured at any point during the patient’s illness, continuously or episodically.[23–32] These characteristics add specificity to the APACHE II model. In an interesting study, Reintam analyzed IAP and APACHE II as independent predictors of death in patients admitted to ICU, using univariate analysis and subsequently adding variables to a multiple regression analysis. He concluded that APACHE II at admission is the most sensitive predictor and that IAP and lactate values are also good independent predictors of mortality.[45]
Our findings suggest that combining APACHE II variables and IAP as prognostic factors in the same model permits a more comprehensive and objective approach to predicting mortality, since their strengths are complementary. From this point of view, the most comprehensive model is APACHE II+IAP, since it has APACHE II’s demonstrated utility in estimating magnitude of severity in the critical patient,[16,18,37] along with IAP, which is very informative about progress in postlaparotomy patients.[44]
Our study has several limitations. It was done in a single facility, with a high volume of reoperated patients and high mortality, which could limit external validity. It must be remembered, however, that these are severely ill patients, with APACHE II scores >13 points, with mortality within permissible limits for the severity of their peritonitis.
All three models demonstrated validity for estimating probability of death in surgery patients treated in the ICU after abdominal surgery; i.e., they are reproducible in populations with similar characteristics. For practical reasons, the authors propose the IAP-based model, which is equivalent to the other two models in discriminative capacity, but is less expensive because it involves just one variable, does not require laboratory tests (like APACHE II), and follows the principle of parsimony, which is crucial in biomedical modeling.[46,47] The IAP-based model is the most economical and therefore the most applicable in conditions of low material and human resources and in contingency and disaster areas.
Clinical judgment is often biased by subjectivity, interindividual variability, lack of reproducibility and tendency to overestimate,[48] since it depends on physician experience, specific skills, and values. In the case of critical patients, psychic stress or case overload are almost always present and can increase the probability of bias when formulating a prognosis. These characteristics justify the need to rely on more objective, reliable and reproducible prognostic tools. Further studies should consider clinical validation of these results in other contexts, and explore automation, which would facilitate use of IAP to assess prognosis at the bedside.
CONCLUSIONS
The three models possess good capacity and similar effectiveness for prediction of discharge condition in MAS patients. Although the model based on APACHE II+IAP is a priori more comprehensive, the IAP model is more feasible, especially in limited-resource conditions.