ABSTRACT
INTRODUCTION To provide equal access, health care provision should be distributed across geodemographic space based on need. In Argentina, the social security, publicly funded health care and private health care subsectors are responsible for delivering health services. In the public subsector, which is responsible for providing primary and secondary care mainly to the uninsured population, supply of services is not always associated with need. The lack of coordination between levels and subsectors makes it difficult to transform need into demand.
OBJECTIVE Design a methodology to systematically estimate need, demand and supply of primary health care services based on secondary data sources in order to assess potential mismatches in any geographical area.
METHODS An ecological analysis was conducted based on outpatient visits in primary care in Bahía Blanca, Buenos Aires Province, Argentina. A mathematical approach was proposed to systematize data collection by census tract regarding estimated need (number of outpatient visits needed, by specialty, according to age- and sex-specific care protocols and the area’s demographics), demand (actual outpatient visits by specialty in each primary health care center), and supply (visit capacity or available appointment slots, taking into account number of personnel hours worked, by specialty).
RESULTS Demand for outpatient visits exceeded need (299,731) by 24% while available visit capacity (993,903) could have covered more than twice the number demanded (370,881). Analysis of the three variables grouped by area found that supply correlated more closely with demand (ρ = 0.90) than with need (ρ = 0.68), while spatial analysis showed that supply distribution responded to need. Areas with greater need had a health facility relatively close by, although supply was often located in areas of lower need, and some areas struggle with relatively high need and insufficient supply.
CONCLUSIONS Results suggest the need for some reconfiguration of primary health care in the study area. The proposed mechanism for estimating relationship among supply, demand and need is a useful tool to support decision-making.
KEYWORDS Health services needs and demand, access to health care; health care accessibility, health care quality, access, and evaluation, health care inequalities, primary health care, Argentina
INTRODUCTION
Primary health care resources should be distributed differentially to ensure equitable population access according to need. To do so requires taking into account many differences (demographic, epidemiological, geographic, sociocultural, economic and political) between groups[1] using information from population censuses, vital statistics, epidemiological studies, studies of individual perceptions, clinical measurements, as well as facility and specialty utilization rates and costs.[2]
Indicators of need must be considered separately from utilization/demand indicators, because not all needs are perceived, and when they are perceived, may not lead to attention at health services because of barriers to access (lack of money, distance, cultural differences between providers and consumers, etc.).[3] Thus, when evaluating access to health care, the dimension of need must be considered along with interactions between supply and demand. An integrated analysis can help identify whether supply adjusts to need and demand, whether need translates into demand, and whether supply falls short of demand.[4]
Methodologies available for these studies include those based on health economics, health planning, and operations research. The first type applies econometric models to study utilization based on observational data. The second applies needs indicators and assesses relative need per capita for services at various government levels by type of service. The third examines optimal use of resources by applying tools such as mathematical programming, simulation and systems dynamics, and, unlike economic or planning methodologies, involves decision-makers.[5]
IMPORTANCE The proposed methodology supports systematic estimation of a population’s health service need, demand and supply in a relatively simple and low-cost way.
This last methodology’s main strength is that it provides input for designing decision-making support tools based on simultaneous analysis of information about the three dimensions of interest (need, demand and supply), information which can be obtained free of cost from available secondary sources. This approach turns out to be highly useful for decision-makers in the health sector who want to optimize resource allocation.
For example, this approach has been used to determine optimal locations for community health care facilities.[6–8] It is especially important to assess where to locate primary health care (PHC) facilities, in Argentina called Primary Health Care Centers (CAPS).[9] CAPS provide less-complex services in public health systems intending to strengthen primary health care.[10] In Argentina, the health system’s subsectors (public, private, and social security) are poorly integrated, leading to many situations of unequal access.[11] Some 38% of the population is uninsured and this group mostly uses the free services provided by the public subsector.[12]
Selection of CAPS locations responds to multiple factors (historic, cultural, economic, political) that can skew supply away from need. This mismatch can be observed in Buenos Aires Province where PHC provision has been delegated to local governments, which has led to extremely unequal access to health services among regions; access ends up responding to differences in economic standard of living rather than differences in need.[13] Nevertheless, available evidence does not suggest such inequitable access within each locality.
Most studies on access to PHC services examine supply and demand through the ratio of providers to population, which can help identify resources available in a given area but does not reveal variation within it.[14–16]
Methodologies need to be designed that can answer these two questions: Is supply in each locality geographically distributed in such a way as to meet demand? To what extent does demand in each area reflect real need? Such analysis calls for information that is difficult to collect, especially in poorly coordinated decentralized health systems or those with substandard information systems. A mechanism needs to be designed to systematically estimate these figures in order to implement decision-making support tools.
Health systems that lack information systems need a protocol for deriving indicators of supply, demand and need that would enable progress toward implementation of systematic decision-making processes based on knowledge of these variables.
In the Bahía Blanca Partido (a subdivision of Buenos Aires Province), public health system managers have expressed concerns about matching PHC supply to need.[17] The Bahía Blanca case study is interesting for several reasons:
- While each CAPS can treat on average about 5500 residents (1500 uninsured)—in line with widely accepted potential access conditions in relation to the estimated national average (1 CAPS for every 3184 uninsured individuals)[18]—it remains unclear whether geographic service distribution responds to regional variation in need.
- In many cases, CAPS operate out of neighborhood development associations (nonprofit organizations that carry out activities to provide community services in the neighborhood and its surroundings). These associations opened their premises to house the CAPS and are opposed to any relocation.
- The partido’s complex situation—with a large population widely dispersed over extensive territory, and many differences evident among census tracts (socioeconomic conditions, demographic features, health and epidemiological risks, etc.)—calls for a mathematical approach.
The purpose of this study was to design a methodology based on secondary data sources to help systematically estimate supply, demand and need in PHC services in order to assess potential mismatches within geodemographic areas. Specific objectives include: design a method to estimate need for PHC outpatient visits based on specific population features; determine demand for PHC visits; quantify supply based on available personnel; and assess the relationship between supply, demand and need for PHC visits in Bahía Blanca.
METHODS
An ecological analysis was conducted to examine supply, need and demand for outpatient visits in seven basic PHC services (nursing, general family medicine, pediatrics, gynecology, obstetrics, mental health and dentistry) in Bahía Blanca Partido in 2015.
Study area Bahía Blanca Partido in Buenos Aires Province, Argentina includes three urban areas, Cabildo, General Daniel Cerri and the city of Bahía Blanca (in the remainder of the text, if Partido is not specified, Bahía Blanca refers to the city). According to Argentina’s National Statistics and Census Institute, Bahía Blanca Partido’s 2010 population was 301,572, 27% without health insurance.[19]
Bahía Blanca Partido’s health system has facilities in the public health subsector at three government levels: municipal, provincial and national. The provincial level is responsible for the Dr José Penna Interzonal General Acute Care Hospital, and the municipal level is responsible for Dr Leónidas Lucero Municipal Acute Care Hospital and 56 CAPS. The CAPS treat less complex health problems and carry out health promotion; they treat mostly uninsured low-income individuals. Of the 56 CAPS, 5 are health centers (HC), which offer a wide range of specialties, and 51 are health units (HU) with reduced services and operating hours. The CAPS are grouped in 11 Program Areas (AP) (based on geographic, demographic, health and technical or administrative factors), each run by a management team.
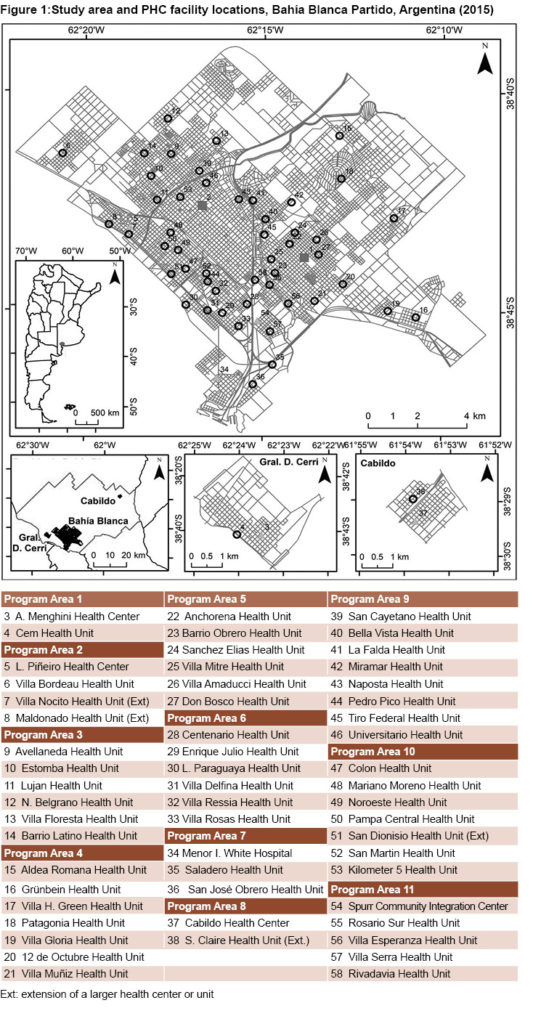
Figure 1 shows the geographic distribution of CAPS in the study area, and the APs to which they belong.
Case study conception, design and application Conception The analysis unit for need was the census tract, a geographically defined space that includes a given number of households. Census tracts can be urban, rural or mixed, depending on population dispersion.[20] Estimates of annual outpatient visits needed per specialty in each census tract were based on size of population requiring checkups and annual frequency recommended for the population group in accordance with clinical practice guidelines and medical protocols established in Argentina.[21–30] Given that these recommendations represent the healthy population’s need for routine care, the estimate was adjusted for conditions in the home in order to capture the potentially greater need associated with social determinants of health.
The CAPS was the analysis unit for quantifying supply and demand. Supply was estimated by measuring the number of visits potentially available per specialty based on annual hours worked by each professional and number of visits per hour that can be accommodated by specialty. Demand was determined by the actual number of visits by specialty in each CAPS.
Although mismatches among need, supply and demand do not necessarily occur in geographic zones and may be due to other factors, this approach (which focuses on geographic accessibility) was used to diagnose the need to relocate CAPS. Data were presented according to AP, since each CAPS belongs to an AP, which comprises a set of census tracts. To assess the relationship among dimensions—supply, demand, and need—the Pearson correlation coefficient, ρ, was calculated for all visits by specialty for demand and supply (by CAPS and AP) and for supply and need (by AP). Correlation thresholds were ρ = 0.9 for very strong, ρ = 0.75 for strong, ρ = 0.5 for moderate, ρ = 0.25 for weak, and ρ = 0.1 for very weak.[31] SPSS 15 software was used. Analytical distribution maps were compiled by geographical zone (for need) and facility (for supply and demand); form, color and size were used as visual representation. The shade of each census tract represented level of need for visits. We used SIG software[32] for classification using the natural breaks method,[33] which identifies maximum homogenization within each classification and maximum difference among classifications, so limits can be established when a relatively significant jump occurs between values.[34,35] CAPS were represented by concentric black and grey circles proportionate to size of the facility’s supply of and demand for visits, respectively.
Design Need. Annual need per census tract for each specialty was estimated based on size and requirements of different population groups (PG) according to age and sex. To capture health needs, the following PGs were defined: aged<1 year, aged 1 year, aged 2 years, aged 3–4 years, aged 5–9 years, girls aged 10–14 years, boys aged 10–14 years, women aged 15–19 years, men aged 15–19 years; women aged 20–39 years, men aged 20–39 years, women aged 40–64 years, men aged 40–64 years, women aged ≥65 years, and men aged ≥65 years. Estimates of need were made with the following equation, in which each census tract’s needs depend on number of individuals in the different population groups () and a set of variables and parameters:

= specialty j visits needed in one year in census tract r
= number of individuals belonging to
in census tract r
= uninsured proportion of 
= proportion of population exposed to adverse living conditions in census tract
= proportion of
needing specialty j
= annual frequency of visits in specialty j needed by 
represents the proportion of uninsured individuals in a PG and is significant because this is generally the only population that demands PHC services in the publicly funded health care subsector. The parameters and represent proportions of the population that would use each specialty according to age and sex, respectively, and number of annual checkups recommended for each PG by specialty. These factors are fundamental inputs to obtain the number of visits needed based on each census tract’s demographic data. Their values are established according to evidence-based medical guidelines for routine care in healthy populations.[21–30] To replicate the study in another area, parameters should be adapted to outpatient visits needed by specialty according to health authorities’ criteria in each jurisdiction, determined by adapting sound international scientific evidence to the local context to derive minimum requirements.
Finally, assuming that adverse socioeconomic conditions can swell the need for health services beyond scientifically based recommended levels for healthy populations, the number of outpatient visits needed in each census tract was affected by parameter , which measures general environmental quality of life. Each census tract’s housing shortage was estimated by the proportion of critically overcrowded households (indicated by the variable overcrowding recorded in the national census: households with >3 individuals per room). Household overcrowding was included as a surrogate for the social determinants of health.[36]
Demand. Annual demand by specialty () was obtained for each CAPS from number of visits registered by the health authority (). Since these figures can vary, the following calculation is proposed:

in which
= specialty j visits demanded in one year in CAPS c
= specialty j visits registered in one year in CAPS c
= correction factor for specialty j demand in CAPS c
0 ≤
≤1
With the addition of factor
, the formula is adjusted for potential over-registration, capturing situations in which the target population’s demand for outpatient visits does not match the number of visits registered by the health authority. Such differences can occur when individuals with health coverage or residents of other municipalities use PHC services in the study area. This factor can vary from one CAPS to another. For example, facilities with greater response capacity and facilities located near the partido’s borders are more likely to attract individuals who are insured or who live in neighboring areas. This factor could also vary by service. It is difficult to obtain in poorly computerized systems and can be estimated based on surveys of CAPS personnel and client population or obtained from health authority registries.
Supply. Annual supply in each CAPS by specialty (
) was calculated based on time available per year and each specialist’s productivity:

in which
= specialty j visits provided in one year in CAPS c
= specialty j visits provided per week
= specialty j hours available per week in CAPS c
= number of weeks per year
To determine the supply (visit capacity), the annual number of available hours was multiplied by a parameter measuring the specialty’s productivity (
) approximated by maximum possible number of visits per hour. According to a literature review, the average time for a clinical outpatient visit is 10–15 minutes,[37] which would give
values of 4–6. This figure may differ from one CAPS to another.
Hours per week by specialty in each CAPS (
) were obtained by multiplying total number of health personnel (nurses, physicians, technicians, etc.) assigned to CAPS c (
) by hours contracted per week (
):

= specialty j professionals in CAPS c
= weekly hours contracted by specialty j professionals
= correction factor for specialty j supply
0 ≤
≤1
The value
assumes a homogeneous format for contracts in which all professionals in a certain specialty are contracted for the same number of hours per week in the CAPS where they work. A more general model could consider the weekly number of hours contracted, which differs from one CAPS to another or even among individual professionals.
Parameter
was also incorporated as a correction factor for outpatient visit capacity per year to consider the amount of time specialists allot to work activities not directly involved in patient care (teaching, research and/or administration) and to more precisely estimate the amount of time effectively available for patient visits. The parameter may vary according to specialty and to CAPS. Its estimated values should be determined based on interviews with health personnel.
Application in Bahía
Blanca Partido Need estimates were made based on population data from the 369 census tracts of the 2010 national census (excluding nine rural census tracts with a total population of 7340). [19] Populations per PG in 2015 were estimated based on 2010 population per census tract adjusted by the average municipal annual population growth rate according to projections for 2015[38] and proportions represented by each PG in 2010. Health coverage for each PG was estimated from municipal and provincial data.[39,40] With respect to nursing services, the maternal and child population was not affected by the correction factor, since it is mandatory for all pregnant women, as well as new mothers and children, to receive services at a CAPS facility for vaccinations (in accordance with the country’s official immunization schedule). Table 1 presents proportions of each PG in total population and percentages of uninsured.
Supply was estimated based on number of professionals assigned to each CAPS, according to whether they have 24- or 40-hourweek contracts with an average visit session lasting 16.7 to 18.5 minutes,[41] an adjusted figure based on expert opinions in the specialty. Finally, due to lack of information on supply and demand correction factors, these were considered to be equal to 1. Data were provided by the Health Se
cretariat of Bahía Blanca Partido.
Need for outpatient visits by specialty was estimated based on the values of and as determined from a literature review (clinical practice guidelines, population studies, utilization rates, etc.). In Table 2, each specialty has two columns: indication for visit and frequency of visit.
All children aged <1 year, for example, should be brought to CAPS for 12 visits with a nurse and 10 pediatric checkups annually. This group does not require treatment from other specialties at PHC levels.
Nursing services included vaccinations according to Argentina’s national schedule.[21] For children and adolescents, information from pediatric and dental services was used as recommended by the Argentinean Pediatrics Society;[22] and for adults, from the regularly scheduled routine medical care of healthy adults.[23,24] For mental health, prevalence of psychiatric disorders was used, as well as proportion of the national population aged ≥15 years affected by mental and behavioral disorders.[25,26] For obstetrics, we assumed the early adolescent (ages 10–14 years) and late adolescent (ages 15–19 years) pregnancy rates for Buenos Aires Province and the mean age-specific fertility rates for women aged 20–39 years; and estimated needed visit frequency according to health authorities’ recommendations for Buenos Aires Province and Argentina regarding antenatal care in low-risk pregnancies.[27–30]
RESULTS
According to 2010 projections, an estimated 299,731 outpatient visits with all PHC specialists treating Bahía Blanca’s uninsured population were needed in 2015 (with the exception of nursing services, which provide treatment for the entire maternal and child population). The population in each census tract needed an average of 812 visits. The census tract with the least need registered 81 visits and the one with the most 3095.
Distribution of need for outpatient visits by specialty included: nursing services (41%), dentistry (25%), pediatrics (17%), gynecology (35%), mental health (4.6%), general family medicine (3%) and obstetrics (2%). However, need for services varied by neighborhood and locality.
Demand was quantified at 418,214 outpatient visits. There were 370,881 visits with specialists (89% of total). Differences among CAPS were significant: CAPS that provide specialties handled 36% of visits and 4 of the 5 HCs accounted for 20% of total visits. Average number of visits per CAPS was 4302 in nursing, 1111 in general family medicine, 518 in pediatrics, 251 dental appointments, 151 in mental health services, 147 in gynecology, and 116 in obstetrics (values varied among CAPS).
Regarding supply, available municipal PHC person-hours in 2015 were sufficient for a total of 993,903 outpatient visits. CAPS provided an average of 10,162 appointments in nursing, 3287 in general family medicine, 1508 in pediatrics, 819 in gynecology, 761 dental appointments, 624 in obstetrics and 558 in mental health services. Important differences were observed among CAPS in outpatient visits by specialty: an average of 3638 pediatric visits in each CAPS providing all specialties (4 HCs and 7 HUs), but only 1110 in CAPS with pediatric services but not all the other specialties (1 HC and 26 HUs).
Based on this protocol, it was estimated that in 2015 Bahía Blanca saw demand exceed need (299,731 visits) by about 24%, while theoretical supply (993,903 visits) was more than twice the number of visits demanded (370,881).
Distribution of need, supply and demand was mapped spatially for each service, For reasons of space, results are presented for only four services (nursing, dentistry, general family medicine, and pediatrics), selected because they revealed different types of results: nursing services accounted for greatest need (41%), supply (57%) and demand (65%); general family practice services accounted for the next greatest supply (19%) and demand (17%) but not need (4%); dentistry presented greater need among services (25%) with insufficient supply to cover it, and pediatrics presented somewhat high need (17%) totally covered by supply.
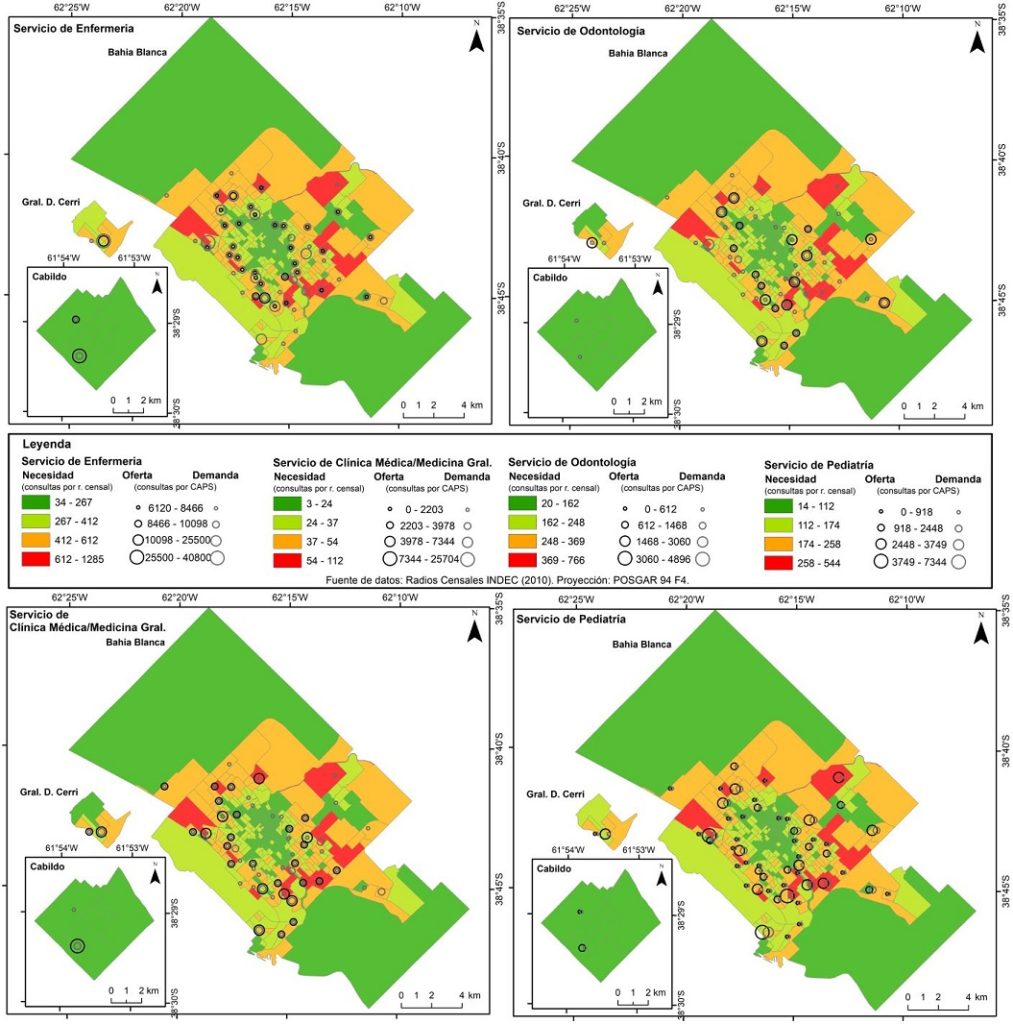
CAPS: primary health care centre | PHC: primary health care
Figure 2 shows that spatial distribution of need is similar among services. The census tracts needing more annual visits coincide for all services and are generally located on the periphery of Bahía Blanca. Supply dispersion increases the further a CAPS is located from the center of the city. The CAPS with greatest supply, however, are not necessarily located in the census tracts with the greatest need.
Most census tracts with greater need had a CAPS nearby, and all five HCs were located in the areas with greatest need. On the other hand, for all services, there were CAPS in low-need census tracts. For all services there were no CAPS nearby in census tracts with mid-level needs.
Table 3 presents distribution of supply, demand, and need for outpatient visits by AP for each specialty.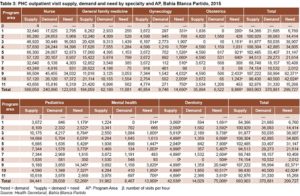
Nursing services were the most readily available and provided 57.3% (569,058) of total visit supply (993,903), followed by general family practice with 18.5% (184,059) and pediatrics with 8.5% (84,441). Over half of total PHC supply was concentrated in five APs: AP #4 with population of 108,304 (10.9%), AP #9 with 107,222 (10.8%), AP#5 with 102,485 (10.3%), AP #2 with 100,929 (10.2%), and AP#10 with 98,430 (9.9%). Each of these APs contained at least one CAPS that provided all specialty services.
With respect to distribution of demand by AP and specialty, it was observed that nursing, general family medicine, and pediatric services accounted for 90% of visits and 65% (240,890), 16.8% (62,189) and 7.8% (29,038) of demand (370,881), respectively. Four APs (AP #9 with population of 56,994 or 15.4%, AP #3 with 50,035 or 13.5%, AP #4 with 42,885 or 11.6%, and AP #10 with 40,500 or 10.9%) accounted for over half of total visits. The CAPS of Cerri (AP #1); White (AP #7) and Cabildo (AP #8) accounted for smaller percentages of visits in all specialties (5.8%, 4.9%, and 2.8%, respectively).
The correlation assessment indicated a positive and strong relationship between supply and demand when analyzed by CAPS as well as by AP, except for general family medicine. In both CAPS and AP analyses, the highest correlation coefficients corresponded to pediatrics (0.929 and 0.951, respectively).
Distribution by AP of outpatient visits needed indicated that those with greater need also presented greater demand. These were: AP #9 with a need for 82,371 visits (27.5%), AP #10 with 42,024 (14%), AP #3 with 38,907 (13%) and AP #4 with 34,805 (11.6%), which together covered 66% of total need (299,727) and 52% of demand (370,881). A strong positive correlation was found be tween demand and need by AP (ρ = 0.90), while the correlation between need and supply was still positive but weaker (ρ = 0.68) and varied according to specialty: considerable for nursing services (ρ = 0.754) and weaker for general family medicine (ρ = 0.252).
Comparison of 2015 visit numbers showed that, except for nursing and general family medicine services, some APs presented greater need than demand. This was observed in all APs for dentistry, the only service in which supply did not match total coverage needed overall, nor by AP (except for AP #7). For total services, AP #9 and AP #10 were the only ones where need for visits exceeded demand. In AP #10, supply exceeded need, which was not true for AP #9.
DISCUSSION
Various methodologies have been used to examine the relationship between supply, need and demand in health services. A study in Canada assessed whether distribution of PHC nurses and physicians matched population needs (measured by standardized mortality rate).[42] A study in Mexico determined that the need for PHC physicians and nurses per inhabitant (estimated based on expert opinions and utilization data) was greater than supply.[43] Murphy estimated drug shortages using a needs-based simulation model, keeping in mind age, sex, health status and service-use rates.[44] Teerawattananon looked at burden of disease associated with reproductive health as a measure of need in order to design a basic package as part of a universal coverage plan.[45] De Graaf-Ruizendaal estimated need for outpatient visits based on census and service utilization data.[46] Barber Pérez assessed need for medical specialists by region, applying international standards for ratios of human resources to population.[47]
A study in Argentina analyzed each CAPS’ geographic accessibility using effective demand (measured by prescriptions filled) and potential demand (estimated by population density of each census tract in the CAPS catchment area), revealing that some areas had unmet need.[48]
Unlike these studies, we proposed to differentiate need from demand by applying mathematical models to enable estimates based on secondary data sources. Mathematical modeling for optimal positioning of health services has antecedents in the literature. In Cuba, a study of physician demand and distribution used an information tool based on the center of gravity method[49] (which minimizes travel distance) to characterize physician demand by assigned population and evaluate allocation improvements. Griffin implemented an optimal allocation model based on health conditions and utilization rates[50] to estimate visits needed in services provided by HCs. But these studies do not consider differences between need and demand,[42,51] or if they do use some concept of need, it is with broad strokes based on population statistics and some characteristics that modify need.[52]
A study in Argentina to quantify the difference between demand and need examined public provision of medications for uninsured diabetic patients. The results indicated that total public supply covers only about one quarter (25%) of treatment need.[53]
The main innovation of our contribution is including medical recommendations in estimates of need for outpatient visits and not utilization rates, in recognition that utilization indicators do not take into account potential obstacles to access. The method can potentially be extended to all specialties and/or practices where it is possible to identify recommendations by PG. The proposed methodology is also easy to implement via spreadsheets and can be replicated in other regions by updating the data sources.
However, several constraints can be identified:
- While in the effort to prevent information bias it would be appropriate to use datasets recorded in the same year, this was not possible due to lack of annual population statistics and unavailability of information on supply and demand in 2010. The decision was thus made to use population projections for 2010 to 2015 (the year of the study), information which is available at the national level.
- Need may be underestimated since only scheduled checkups are considered, while a significant proportion of outpatient visits in CAPS are spontaneous visits associated with common health problems requiring quick solutions. The difference between need and demand can be expressed by including a correction factor for demand based on estimates of the proportion of this type of outpatient visit.
- Estimates of need are based on PHC clinical practice guidelines for checkups for the healthy population in the study area. Although it was proposed that visit indication and frequency for each specialty follow recommendations based on independently developed evidence, it is recognized that their applicability to other geodemographic contexts would require adapting care protocols to local features, such as, for example, the local epidemiological profile.
- Although CAPS are expected to serve as the entry gateway to the health system, a proportion of the population seeks out a hospital for a problem that could be appropriately resolved in a PHC facility. This factor could be captured by including as units of analysis hospital ambulatory care clinics that provide these specialty services.
- Estimates of service supply should take into account the amount of time professionals are available to see patients and the amount of time dedicated to additional activities (such as administration) in the CAPS. This factor could explain the wide mismatch observed between supply and demand.
- Application of supply and demand correction factors that have theoretically been determined would require fieldwork that exceeds the scope of the present study. For this reason, these factors were applied with a value of 1. However, they could be estimated based on secondary data sources or information from health authorities. This article is the partial result of a larger study to develop a mathematical model for redesigning PHC.
CONCLUSIONS
The proposed methodology enables relatively simple, systematic, low-cost estimation of a population’s health care needs, supply and demand. Such information is essential for making decisions about PHC design in the community and is often difficult to obtain directly in large or poorly computerized systems.
In the case of Bahía Blanca, where some areas were observed to have relatively high need yet no CAPS nearby, the findings indicated the appropriateness of redesigning supply of several PHC services to more closely match distribution to estimated need.
The tool can be replicated in any geodemographic area and applied over time as the variables that determine need, supply and demand change. It has the capacity to predict future discrepancies and can therefore be widely used in PHC services planning.
A future line of investigation is to modify the methodology to calculate precise values for the proposed correction factors.
References

- Bani Health needs assessment. J Family Community Med. 2008 Jan;15(1):13–20.
- Salinas Martínez AM, Muñoz Moreno F, Barraza de León AR, Villarreal Ríos E, Nuñez Rocha GM, Garza Elizondo ME. Necesidades en salud del diabético usuario del primer nivel de atención. Salud Pública Méx. 2001 Jul–Aug;43(4):324–35. Spanish.
- Barragán HL. Necesidades, demanda y oferta de atención médica. In: Fundamentos de Salud Pública. La Plata: Editorial de la Universidad Nacional de la Plata; 2007. p. 353–60.
- Wright J, Williams R, Wilkinson JR. Development and importance of health needs assessment. BMJ. 1998 Apr 25;316(7140):1310–3.
- Kunc M. Modeling supply, demand and need: a literature review. Technical paper series No. 0013 [Internet]. London: Centre for Workforce Intelligence; 2015 [cited 2017 Apr 23]. Available from: http://webarchive.nationalarchives.gov.uk/20160406094904/http://www.cfwi.org.uk/publications/technical-paper-modelling-supply-demand-and-need-a-literature-review
- Daskin MS, Dean LK. Location of health care facilities. In: Brandeau ML, Sainfort F Pierskalla W, editors. The Handbook of OR/MS in Health Care: A Handbook of Methods and Applications. Alphen aan den Rijn (NL): Kluwer; 2004. Chapter 3. p. 43–76.
- Verter V, Lapierre SD. Location of preventive health care facilities. Ann Operations Res. 2002 Feb;110(1–4):123–32.
- Zhang Y, Berman O, Marcotte P, Verter V. A bilevel model for preventive healthcare facility network design with congestion. IIE Transactions. 2010 Oct 9;42(12):865–80.
- Brambilla A, Maciocco G. Le case della salute. Recenti Prog Med. 2014 Apr;105 (4):147–50. Italian.
- Starfield B, Shi L, Macinko J. Contribution of primary care to health systems and health. Milbank Q. 2005;83(3):457–502.
- Belló M, Becerril-Montekio VM. Sistema de salud de Argentina. Salud Pública Méx. 2011 Jan;53(Suppl 2):S96–108.
- Ministry of Health (AR). Encuesta de Utilización y Gasto en Servicios de Salud. Serie 10 No. 21. Argentina-Año 2010. Primeros Resultados [Internet]. Buenos Aires: Ministry of Health (AR); 2012 Jul [cited 2017 Feb 12]. 27 p. Available from: http://deis.msal.gov.ar/wp-content/uploads/2016/01/Serie10Nro21.pdf.
- Lago FP, Elorza ME, Moscoso NS, Ripari NV. Equidad en el acceso a los servicios de Atención Primaria de Salud en sistemas de salud descentralizados: el caso de la provincia de Buenos Aires, Argentina. Rev Gerencia Políticas Salud. 2013 Jul–Dec;12(25):40–54. Spanish.
- Dewulf B, Neutens T, De Weerdt Y, Van de Weghe N. Accessibility to primary health care in Belgium: an evaluation of policies awarding financial assistance in shortage areas. BMC Fam Pract.2013 Aug 22;14:122.
- Gao F, Kihal W, Le Meur N, Souris M, Deguen S. Assessment of the spatial accessibility to health professionals at French census block level. Int J Equity Health. 2016 Aug 2;15:125.
- Amorim Lopes M, Santos Almeida A, Almada-Lobo B. Handling healthcare workforce planning with care: where do we stand? Hum Resour Health. 2015 May 24;13:38.
- Corradetti C. Bahía Blanca se debe reorganizar en salud pública. La Nueva Provincia [Internet]. 2017 Sep 22 [cited 2018 Mar 30]. Available from: http://www.lanueva.com/nota/2017-7-22-9-0-0–bahia-blanca-se-debe-reorganizar-en-salud-publica.
- Stolkiner A, Comes Y, Garbus P. Alcances y potencialidades de la Atención Primaria de la Salud en Argentina. Ciênc Saúde Colet. 2011 Jun;16(6):2807–16. Spanish.
- National Institute of Statistics and Census (AR) [Internet]. Buenos Aires: INDEC; c2002–2014. Base de datos REDATAM. Cuestionario ampliado 2010; [cited 2016 Mar 27]. Available from: http://200.51.91.245/argbin/RpWebEngine.exe/PortalAction?&MODE=MAIN&BASE=CPV2010A&MAIN=WebServerMain.inl.
- National Institute of Statistics and Census (AR) [Internet]. Buenos Aires: INDEC; c2002–2014. Unidades Geoestadísticas. Cartografía y códigos geográficos del SEN; 2015 [cited 2017 Oct 15]. Available from: http://geoservicios.indec.gov.ar/codgeo/index.php?pagina=definiciones.
- Ministry of Health (AR). Calendario Nacional de Vacunación de la República Argentina 2016 [Internet]. Buenos Aires: Ministry of Health (AR); c2018 [cited 2015 Jan 12]. Available from: http://www.msal.gob.ar/images/stories/ryc/graficos/0000000628cnt-2016_calendario_vacunacion.pdf.
- Sociedad Argentina Pediátrica (2012). Libreta de Salud [Internet]. Buenos Aires: Sociedad Argentina Pediátrica; [cited 2015 Nov 15]. Available from:www.sap.org.ar/docs/profesionales/libreta_salud_sap.pdf. Spanish.
- Ministry of Health (AR). Presidencia de la Nación. Estrategia Nacional de Prevención y Control de Enfermedades. Crónicas no Transmisibles. Componente: Servicios de Salud Manual para el Cuidado Integral de Personas Adultas en el Primer Nivel de Atención [Internet]. Buenos Aires: Ministry of Health (AR); c2016 [cited 2015 Oct 20]. Available from:www.msal.gob.ar/images/stories/bes/graficos/0000000816cnt-2016-04_manual-para-el-cuidado-integral-de-personas-adultas.pdf. Spanish.
- Zepeda CAJ. El examen médico periódico del adulto asintomático. Rev Med Hondur. 2011;79(2):94–7. Spanish.
- Ministry of Health (AR). Información básica de salud mental y adicciones infanto-juvenil [Internet]. Buenos Aires: Ministry of Health (AR); 2014 [cited 2015 Oct 24]. 14 p. Available from: http://www.msal.gob.ar/saludmental/images/stories/info-equipos/pdf/2014-12-18_informacion-basica-en-salud-mental-adicciones-infanto-juvenil-2014.pdf.
- Ministry of Health (AR). Estimación de la población afectada de 15 años y más por trastornos mentales y del comportamiento en Argentina 2010 [Internet]. Buenos Aires: Ministry of Health (AR); 2010 [cited 2015 Nov 10]. Available from:www.msal.gob.ar/saludmental/images/stories/info-equipos/pdf/1-estimacion-de-la-poblacion-afectada.pdf. Spanish.
- Ministry of Health (AR). Dirección de Estadísticas e Información de Salud. Boletín 128: Indicadores seleccionados de salud para población de 10 a 19 años. República Argentina. Año 2008. Tasas de fecundidad adolescente, temprana y tardía por cada 1.000 mujeres adolescentes según jurisdicción de residencia de la madre [Internet]. Buenos Aires: Ministry of Health (AR); 2010 [cited 2015 Dec 10]. 65 p. Available from: http://deis.msal.gov.ar/wp-content/uploads/2016/01/Boletin128.pdf.
- Sacco N. Evaluación general de los datos de fecundidad del Censo 2010. XIII Congreso de la Asociación Argentina de Estudios de Población [Internet]. Salta (AR): Universidad Nacional de Salta (AR); 2015 Sep [cited 2015 Nov 2]. 24 p. Available from: http://www.economicas.unsa.edu.ar/web/archivo/otros/AEPA/SESION-6/Sesion-6-Sacco.pdf.
- Buenos Aires Province Ministry of Health (AR). Guía de procedimientos para el control del embarazo y la atención del parto y puerperio de bajo riesgo [Internet]. Buenos Aires: Ministry of Health (AR); 2012 [cited 2015 Oct 31]. 136 p. Available from: http://www.ms.gba.gov.ar/sitios/tocoginecologia/files/2014/04/Guia-Control-de-Embarazo-Parto-y-Puerperio-de-bajo-riesgo.pdf.
- Ministry of Health (AR). Recomendaciones para la Práctica del Control Preconcepcional, Prenatal y Puerperal [Internet]. Buenos Aires: Ministry of Health (AR); 2013 [cited 2015 Nov 14]. Available from: http://www.msal.gob.ar/images/stories/bes/graficos/0000000158cnt-g02.control-prenatal.pdf.
- Hernández Sampieri R, Fernández Collado C, Baptista Lucio P. Metodología de la Investig ación. 5th ed. México: McGraw-Hill Interamericana. 2010. p. 311–4. Spanish.
- ArcGis Pro [Internet]. Los Angeles: ArcGIS Pro; c2018. Data classification methods; [cited 2018 Apr 6]. Available from: http://pro.arcgis.com/es/pro-app/help/mapping/symbols-and-tyles/data-classification-methods.htm
- Jenks GF. The Data Model Concept in Statistical Mapping. Int Yearbook Cartography. 1967;7:186–90.
- Buzai GD, Baxendale CA. Análisis socio espacial con sistemas de información geográfica. Buenos Aires: Lugar Editorial; 2006. Spanish.
- Departamento de Asuntos Económicos y Sociales. División de Estadísticas. Estudio de Métodos. Manual de Sistemas de Información Geográfica y Cartografía Digital. Serie F. No.79. New York: United Nations; 2000. 225 p. Spanish.
- Bonnefoy X. Inadequate housing and health: an overview. Int J Environ Pollution. 2007;30(3–4):411–29.
- Outomuro D, Actis AM. Estimación del tiempo de consulta ambulatoria en clínica médica. Rev Med Chil. 2013 Mar;141(3):361–6. Spanish.
- Provincia de Buenos Aires. Proyecciones de población por partido 2010–2025 [Internet]. La Plata: Dirección Provincial de Estadísticas, Buenos Aires; 2015 [cited 2015 May 10]. Available from: http://www.ec.gba.gov.ar/estadistica/Poblacion%20por%20partido%202010-2025.pdf.
- National Institute of Statistics and Census (AR). Censo Nacional de Población, Hogares y Viviendas 2010. Provincia de Buenos Aires, Partido Bahía Blanca. Población total por sexo e índice de masculinidad, según edad en años simples y grupos quinquenales de edad. Año 2010 [Internet]. Buenos Aires: INDEC; 2010 [cited 2015 Apr 5]. Available from:www.indec.gov.ar/ftp/censos/2010/CuadrosDefinitivos/P2-D_6_56.pdf. Spanish.
- National Institute of Statistics and Census (AR). Censo Nacional de Población, Hogares y Viviendas 2010. Provincia de Buenos Aires. Población en viviendas particulares por tipo de cobertura de salud, según sexo y grupo de edad. Año 2010 [Internet]. Buenos Aires: INDEC; 2010 [cited 2015 May 30]. Available from: http://www.indec.gov.ar/ftp/censos/2010/CuadrosDefinitivos/P12-P_buenos_aires.pdf. Spanish.
- Paganini JM, Etchegoyen GS, Bo A, Rubio AM, Stival JJ, Fredeimberg A, et al. Evaluación de sistemas de salud y la estrategia de APS. Rev Argentina Salud Pública. 2010;1(2):18–23. Spanish.
- Wong ST, Watson DE, Young E, Mooney D. Supply and distribution of primary healthcare registered nurses in British Columbia. Healthc Policy. 2009 Nov;5 Spec No:91–104.
- Alcalde-Rabanal JE, Bärnighausen T, Nigenda-López G, Velasco-Mondragón HE, Sosa-Rubí SG. Profesionales necesarios para brindar servicios de prevención y promoción de la salud a población adulta en el primer nivel. Salud Pública Mex. 2013 May–Jun;55(3):301–9. Spanish.
- Tomblin Murphy G, MacKenzie A, Guy-Walker J, Walker C. Needs-based human resources for health planning in Jamaica: using simulation modelling to inform policy options for pharmacists in the public sector. Hum Resour Health. 2014 Dec 6;12:67.
- Teerawattananon Y, Tangcharoensathien V. Designing a reproductive health services package in the universal health insurance scheme in Thailand: match and mismatch of need, demand and supply. Health Policy Plan. 2004 Oct;19 Suppl 1:i31–9.
- De Graaf-Ruizendaal WA, de Bakker DH. The construction of a decision tool to analyse local demand and local supply for GP care using a synthetic estimation model. Hum Resour Health. 2013 Oct 27;11:55.
- Barber Pérez P, González López Valcárcel B, Suárez Vega R. Oferta, demanda y necesidad de médicos especialistas en Brasil. Proyecciones a 2020 [Internet]. Las Palmas: Universidad de Las Palmas de Gran Canaria; 2011 [cited 2016 Mar 24]. Available from: http://www.sbmfc.org.br/media/file/pdf/oferta%20demanda%20y%20necesidad%20de%20medicos%20especialistas%20brasil_patricia_beatriz.pdf.
- De Pietri D, Dietrich P, Mayo P, Carcagno A, de Titto E. Indicadores de accesibilidad geográfica a los centros de atención primaria para la gestión de inequidades. Rev Panam Salud Pública. 2013 Dec;34(6):452–60. Spanish.
- Rodríguez Sánchez Y, Gómez Figueroa O, Diéguez Matellán E, De León Rosales L, Rodríguez González L. Localización-asignación de los servicios de atención primaria en un área de salud. Rev Méd Electrón [Internet]. 2016 Nov–Dec;38(6):837–50. Spanish.
- Griffin PM, Scherrer CR, Swann JL. Optimization of community health center locations and service offerings with statistical need estimation. IIE Transactions. 2008 Jul 17;40(9):880–92.
- Ozegowski S, Sundmacher L. Understanding the gap between need and utilization in outpatient care. The effect of supply-side determinants on regional inequities. Health Policy. 2014 Jan;114(1):54–63.
- García Fariñas A, Ramos Valle I, García Rodríguez JF, Gálvez González AM. El balance entre la oferta y la demanda en salud. El caso de los servicios de rehabilitación integral en La Habana, Cuba. 2009–2010. INFODIR [Internet]. 2011 [cited 2018 Feb 12];7(12). Available from:http://www.medigraphic.com/cgi-bin/new/resumenI.cgi?IDARTICULO=49386. Spanish.
- Elorza ME, Moscoso NS, Ripari NV. Evaluación de políticas públicas de provisión de fármacos para diabetes mellitus tipo 2 en Argentina: estudio de caso. Salud Colectiva. 2012 Jan–Apr;8(1):35–45. Spanish.
THE AUTHORS
María E. Elorza (Corresponding author: meugilorz@hotmail.com), economist, Southern Economic and Social Research Institute (IIESS), National Research Council and Southern National University (CONICET–UNS), Bahía Blanca, Argentina.
Nebel S. Moscoso, economist, IIESS, CONICET–UNS, Bahía Blanca, Argentina.
Aníbal M. Blanco, chemical engineer, PLAPIQUI Institute, CONICET–UNS, Bahía Blanca, Argentina.
Jorge O. Gentili, geographer, Department of Geography and Tourism, CONICET–UNS, Bahía Blanca, Argentina.
Submitted: August 11, 2017 Approved: July 05, 2018 Disclosures: None