INTRODUCTION
Context is widely used in various areas of knowledge, commonly referring to political, historical, sociocultural, linguistic, health and other domains. All human activities are associated with and conditioned by context, their meaning and importance assessed differently depending on the context in which they appear.[1,2].
Contextual factors are expressed in several interrelated geographic dimensions, from national to local levels. In each dimension, processes occur with specific dynamics that influence other hierarchical dimensions. Thus, the national level imposes the macrostructure determining economic and political characteristics and the population’s general living conditions, as well as basic principles of social organization: equality of rights, equity, equitable access to services, and nonexclusion. Processes at the local level occur in the community, where members of a defined population live in the same area and therefore share similar conditions of the physical and social environment in which they carry out their daily activities.[3]
Contextual factors—such as local political organization, social solidarity networks and public service infrastructure in urban areas—are measured at the aggregate, not individual, level. Cities, for example, constitute a geographic space in which numerous common factors link people in complex interrelated webs, although these may not show clear spatial representation and may be difficult to detect.[1,4,5] Although health events occur in individuals, who become ill, receive attention to their needs (or not), and die; the determinants of such events operate outside and beyond them, at these contextual levels.[3]
Various researchers have debated theoretical conceptions and conceptual model definitions that explain the collective processes determining health. Some of these models are noteworthy, as they systematically display the implications of studying contextual factors and have significantly contributed to methodologies for community-based research. Some authors have identified various aspects of the physical and social environment that can promote or compromise health, such as:[1,4–6]
- physical environmental conditions shared by all residents in a location (e.g., air and water quality);
- availability of healthy environments in the home, at work and in recreational areas;
- services that support daily activities (education, transport, waste management, street lighting);
- local sociocultural resources (political, religious and ethnic history; degree of community cohesion); and
- the area’s reputation (as perceived by residents and public administrators).
Ecological variables affect health events, independent of individual characteristics, and also may modify the way in which individual characteristics influence health.
Ecologically oriented, epidemiology, may be useful for identifying social contexts in which disease occurs, is diagnosed and managed.
Abundant recent evidence points to important effects of physical surroundings on health. These are expressed in different spheres, such as quality of life in the elderly, population lifestyles, health perceptions, child welfare, violence, and specific mortality and morbidity rates.[7–11]
Neighborhoods are not passive resource depositories, but rather relational structures, never independent from their residents’ practices. Access to resources is determined by rules of interaction, such as proximity (persons living in the same area share the same physical environment and are exposed to basically the same positive and negative influences); prices (regulated to varying degrees by the market); rights (institutionally governed); and by informal reciprocity. These four ways of interaction operate in five domains specific to the community environment: physical, economic, institutional, local social life and community organization.[6]
Contextual factors’ effects do not only express themselves in intrinsically ecologic conditions—such as environmental contamination or climate—that affect all individuals to a greater or lesser extent. They also express themselves in social circumstances such as poverty: those who reside in a poor community will be affected by all its consequences—such as violence, lack of community hygiene or precarious services—independent of personal economic status.[12,13]
The study of context has tended to focus on demonstating its direct or indirect effects on health indicators, but there have been few attempts to examine its mediation of other factors’ effects. Although individual chronic disease risk factors are reasonably well known, the same is not true of the complex of factors operating at different environmental levels, from home to community.
This study attempts to estimate the relative influence of contextual factors compared to individual factors as determinants of morbidity from type 2 diabetes and heart disease. These conditions have been selected because: they fall within the research mandate of the Atherosclerosis Research and Reference Center (CIRAH, the Spanish acronym) in Havana; heart disease has been the main cause of death in Cuba and in Havana for several decades; and diabetes is the eighth cause of death in Cuba, and the seventh in Havana.
Cuba’s health system is organized on principles of equity, equality of rights, universality and nonexclusion. Primary health care is organized into community polyclinics, each serving as a hub and referral center for a given number of neighborhood family doctor-and-nurse offices (CMF, the Spanish acronym). These offices, in turn, serve a defined population (in urban areas, currently about 1200 persons) residing in a given neighborhood within the community. The family doctor usually lives in an apartment above his/her office. These CMF catchment areas, or neighborhoods, are the setting for our research.
METHODS
A cross-sectional study was carried out at individual and aggregated levels in families registered in 12 CMFs assigned to seven Havana polyclinics. CMFs were purposefully selected based on feasibilty—their family doctors were collaborators in the research—to facilitate optimal response rate. There was no presumption of municipal or provincial representativity.
From these CMFs, 840 families (70 from each CMF) were selected by simple ramdom sampling. Sample size needed was estimated based on the following considerations:
- a main factor whose effect was to be assessed and specific damage defined by a disease arising as a result of atherosclerosis;
- damage present in 20% of the population and an odds ratio of 1.5 as the minimum detectable effect, with α = 0.005 and power 80%; and
- a set of control variables with a positive rate of 30% in cases and with a predictive power of 20%.
An ample estimate of 30% loss or nonresponse was added to sample size and rounded up to the nearest 10 subjects.
The context described and analyzed in this research is represented by the household and neighborhood/CMF. By household, we mean a set of objective and subjective variables that determine features of housing conditions and certain aspects of family perceptions of their own economic and health status. By neighborhood/CMF, we refer to certain aggregate attributes that characterize not the CMF itself, nor its services, but rather the CMF geographic catchment area.
Through home visits, a survey was taken of family members aged ≥20 years, in order to collect data on socioeconomic variables (age, sex, years of education completed), living conditions, perceived economic status, morbidity and risk factors. Physical measurements were taken by family doctors.
Living conditions assessed were:
- unsafe housing (severe building deterioration and/or declared uninhabitable by the housing authority, improvised or built with inadequate materials);
- housing structural problems (roof leakage, loosened or collapsed roof, shored-up or cracked walls);
- overcrowding (overcrowding index was calculated by dividing the number of persons habitually sleeping in the house by number of rooms used for sleeping. Result of >2 defined as overcrowding);
- water supply (presence or absence of running water);
- necessary household appliances (refrigerator, television or radio, fan, iron, pressure cooker);
- secondary household appliances (any not included in the previous list and not essential for satisfaction of basic daily-life needs);
- cooking fuel (gas main, refillable tank, kerosene, electricity); and
- perceived economic status (excellent, good, fair, bad or very bad; as defined by perceptions of all individuals in household aged ≥20 years; most and least favorable perceptions were recorded; occasionally these coincided).
Physical measurements included weight, height, body mass index (BMI, low <18.5, normal 18.5–24.9, overweight 25.0–29.9, obese >30); waist circumference (normal for women ≤80 cm, for men ≤90 cm, per WHO), waist-to-hip ratio (normal for women ≤0.80, for men ≤0.95).
Individual risk factors and health conditions included smoking; exposure to secondhand smoke; diagnosed heart disease (ischemic cardiopathy, cardiac failure, arrhytmia and heart attack), type 2 diabetes or hypertension.
Household density of diabetes and heart disease was calculated by dividing the number of patients by the total number of persons aged ≥20 years in the household. The neighborhood/CMF mean was the arithmetic average of household densities in the catchment area population. It is important to note that density, unlike more usual measures such as incidence or prevalence, describes family characteristics and therefore suggests the degree of family aggregation of population morbidity.
Principal components analysis (PCA) was applied to the matrix of correlations between variables at the three levels (individual, household and neighborhood/CMF) to obtain a minimal set of mutually-uncorrelated indicators capturing the essential features of each level. Correlation rather than the usual covariance matrix was used, due to the different scales on which the variables are measured, especially at the household level. The variables specified for inclusion at each level were:
At neighborhood/CMF level, household averages for:
- Highest educational level completed
- Most unfavorable perceived economic status
- Most favorable perceived economic status
- Secondary household appliances
At household level:
- Overcrowding
- Necessary household appliances
- Secondary household appliances
- Running water
- Unsafe housing
- Housing structural problems
- Cooking fuel
At individual level:
- Perceived economic status
- Waist circumference
- Waist-to-hip ratio
- Highest educational level completed
- Nutritional status (as measured by BMI)
- Smoking
PCA is a data simplification procedure; it replaces a large group of original variables with a smaller group of new variables called principal components, which are synthetic, mutually independent variables that condense a large proportion of the relevant information. The first component explains most of the total variance and the remaining ones, most of the residual variance. PCA is useful for exploratory data analysis, later performing other analyses based on its components. In this study PCA is merely used as an instrumental resource.
Subsequently, contextual logistic models were applied with a nested-model strategy to identify direct and mediating effects of explanatory variables at individual, household and neighborhood/CMF levels. Fitting these models permitted estimation of the relative contributions of neighborhood and household effects on diabetes and heart disease versus those of individual risk factors.
The study received ethical and scientific approval by CIRAH’s scientific council. Written informed consent was obtained from all participants.
RESULTS
Household density for both diseases was similar for all neighborhoods/CMFs combined: 8.6% for diabetes and 8.7% for heart disease, but substantial variation appeared across neighborhoods. There were 3 neighborhoods/CMFs with diabetes density of ≥10%; the highest, 13.4%, was recorded in CMF No. 4. For heart disease, the highest densities recorded—in CMF No. 1, 13.5% and CMF No. 3, 13.3%—were over triple the lowest density, 4.1% in CMF No. 11 (Table 1).
Table 1: Mean household diabetes and heart disease density by neighborhood/CMF
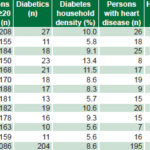
CMF: Family doctor-and-nurse office
Table 2 summarizes PCA results, showing for each level the percentage of variance explained by component and the original variables’ factor loadings for components.
The first component at the neighborhood/CMF level (which is named f1_cmf) accounts for almost 63% of variability. This component depends (in order of contribution) on secondary household appliances, least favorable perceived economic status and educational level completed. A high score for this synthetic indicator will be found among residents in neighborhoods/CMFs where there is a high average number of secondary household appliances, where there is a high average least favorable perceived economic status, and where average educational level completed is high.
At the household level, three components were obtained that explain almost 60% of variability. The components are named f1_hou, f2_hou and f3_hou. A high score for the first component characterizes households where housing is generally precarious, with both safety and structural problems exerting a strong influence. The second and third components are marginal components, accounting for only 16% and 14% of variability, respectively. Nevertheless, each describes specific fundamental housing deficiencies.
Table 2: Principal components and factor loadings for neighborhood, household and individual levels
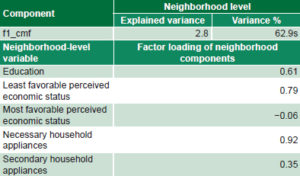
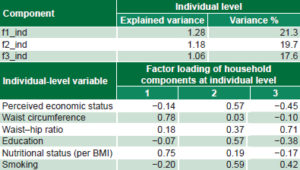
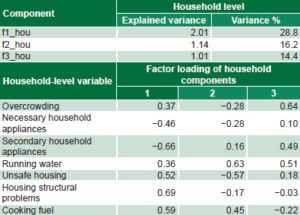
BMI: Body mass index
At the individual level, three components were obtained, which combined account for about 60% of variability, called f1_ind, f2_ind and f3_ind. There is a loose pattern of association among individual variables, as shown by the relatively small variance explained by each component. However, the first component is clearly dominated by obesity and overweight (obese and centrally obese subjects scoring high). The second component is dominated by educational level completed, perceived economic status and nonsmoking; and the third, arguably by android-pattern obesity (high scores with high waist-hip ratios).
In the case of diabetes, as shown in Table 3, the contextual influence of the neighborhood/CMF is modified when household effect is removed (arrows are read as “becomes”): 0.014→ 0.086→ 0.088→ 0.034. The neighborhood effect is indirect, mediated by household, but with very marginal influence; individual coefficients are practically unchanging: 0.157→ 0.159→ 0.158 for the first component; 0.201→ 0.159→ 0.201 for the second, and 0.031→ -0.029→ -0.030 for the third. The principal effect of household (f1_hou) changes noticeably when individual effects are removed, but it is a very weak effect (-0.128→ -0.060→ -0.132). The effect of age is constant. The resulting structural model is represented in Figure 1 (in this and in Figure 2, the dotted line represents the modifying influence of neighborhood on individual factors).
Table 3: Estimated coefficients for 6 logistic regression models nested strategy) for diabetes
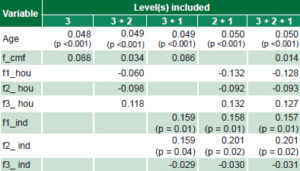
Level 3: neighborhood/CMF Level 2: household Level 1: individual CMF: Family doctor-and-nurse office
Figure 1: Structural model of relative contributions of hierarchical levels as determinants of individual diabetes risk
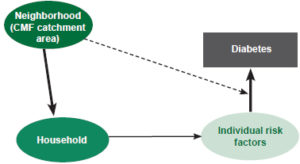
Based on Table 3. Width of arrows roughly proportionate to size of effect; dotted arrow represents modifying effect of neighborhood on individual factors.
CMF: Family doctor-and-nurse office
As can be seen in Table 4, the neighborhood effect for heart disease is slightly modified when household effects are controlled for, but is weak (0.039→ 0.056→ 0.061→ 0.060). Individual coefficients show little change: this may be because hypertension as an individual factor absorbs almost all the effect of the remaining individual risk factors (0.144→ 0.140→ 0.148 for the first component, 0.176→ 0.206→ 0.176 for the second and -0.055→ -0.021→ -0.052 for the third).
There is an important household effect (f2_hou) (-0.267→ -0.265→ -0.264), but this is not modified when individual effects are controlled for, which implies a direct household effect on heart disease risk. Age and high blood pressure coefficients are almost unchanging. The structural model is presented in Figure 2.
Table 4: Estimated coefficients for six logistic regression models(nested strategy) for heart disease
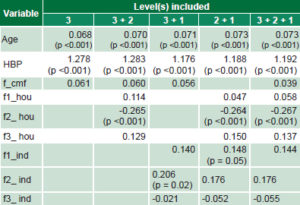
Level 3: neighborhood/CMF Level 2: household Level 1: individual CMF: Family doctor-and-nurse office HBP: hypertension
Figure 2: Structural model of relative contributions of hierarchical levels as determinants of individual heart disease risk
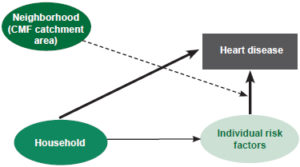
Based on Table 4. Width of arrows roughly proportionate to size of effect; dotted arrow represents modifying effect of neighborhood on individual factors.
CMF: Family doctor-and-nurse office
DISCUSSION
Our results permit us to propose models that must be considered preliminary, since no optimal characterization of the neighborhood/CMF level is available; to approximate this description, we used variables aggregated from the household level. There is no record of this approach being used in other Cuban studies. The structural components of these models are the neighborhood/CMF and household (as context); individual risk factors and conditions, and the dependent variables (diabetes and heart disease), also at the individual level.
Overall (with particular features for each dependent variable), we were able to establish that context exhibits direct effects, mediated effects and interactions with lower contextual levels (i.e., neighborhood/CMF and household) and with individual levels. And we made some progress in quantifying the relative importance of each of these effects.
The classical strategy using nested models provides useful information. Our results are fairly consistent with the volumes of scientific literature regarding contextual determinants generated by interest in the social determinants of health in the last two decades, particularly in developed countries.[14–24] A conclusion common to this research and ours is that, independent of its direct impact on health, an adverse social and physical environment can modify the effect of individual factors. Risk factors do not appear as a result of absolutely free choices made by individuals over their lifespan but are constrained by the physical and social environment. The influence of context, thus conceived, makes itself felt in practically all diseases, with different intensities, but almost always—with rare exceptions—with the socioeconomic gradient in the same direction.
This study identified direct effects of context on health (via various levels of exposure to risk factors) and its modulating effects, not only on individual risk factors, but also on the relationships between these and household and neighborhood. Context affects not only exposure, but vulnerability to exposure.
Understanding disease context and its influence supports planning of appropriate control actions, health promotion and resource allocation. Population health is determined by a group of factors that operate simultaneously at different levels of organization, from individual to social. History, society, culture, family, physical and social environments, and health system organization (all factors contributing to living conditions), as well as lifestyles over the lifespan, determine health status and its population distribution. This has also been shown in other studies.[25–28]
This study has several limitations due lack of knowledge, particularly in Cuba (where the problem has been scarcely studied), of the mechanisms and causal web through which environmental factors influence health. There is, of course, a general conception derived from social epidemiology; but no theory sufficiently developed to facilitate identification of all operating factors, much less quantitative assessment of their importance.
Nor, in terms of methodology, is there an adequately validated metric for important constructs at contextual levels: hence, the tentative use of indicators to characterize households and neighborhoods/CMFs. Nevertheless, our research contributes clues (for example, the importance of perceived economic status) and suggests the need to identify structural contextual factors in followup research.
The study also contributes some quantitative elements to assessment of contextual influences on individual risk of diabetes and heart disease. Interpretation of components is highly subjective; yet, the relative role played by these synthetic indicators at different hierarchical levels (individual, household and neighborhood) were demonstrably important as determinants of diabetes and heart disease at the individual scale.
CONCLUSIONS
We confirmed interactions between individual and contextual (neighborhood and household) factors, whose effects on individual health are not entirely mediated by individual factors. Research needs to pay more attention to context beyond its direct effect on individual risk factors.